Recently, the paper - Highly accurate protein structure prediction with AlphaFold from DeepMind was published in Nature, which unveils the optimized source code of AlphaFold and describes its design framework and training methods in details. Criss-Cross Network (CCNet) proposed by the research team led by Professor Liu Wenyu and Associate Professor Wang Xinggang is one of the important modules of the latest version of AlphaFold. According to the paper, “CCNet and Axial-deeplab have inspired the exploration of attention-based methods for interpreting protein sequences”.
AlphaFold is able to predict 98.5% human protein structure and has increased the prediction accuracy to atom. However, for the past decades, scientists can only predict 17% amino acid residue of human protein sequences. Because of AlphaFold’s great application potential in life science, Shi Yigong, academician of China Academy of Science, spoke highly of AlphaFold, “It is the greatest contribution that artificial intelligence has made to science, and is also one of the most important scientific breakthroughs human beings have made in 21st century. It is a great milestone in the scientific exploration of nature”.
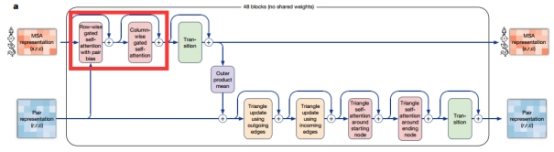
Figure 1: The multiple sequence alignment (MSA) learning module in AlphaFold, in which, the “row-wise self-attention + column-wise self-attention” block proposed in CCNet is stacked for 48 times.
Huang Zilong, a doctoral student of EIC, whose supervisor is Professor Liu Wenyu and associate-supervisor is Wang Xinggang, is the first author of “CCNet: Criss-Cross Attention for Semantic Segmentation”, and Wang Xinggang is the corresponding author. The paper was published on arXiv in 2018, and was accepted by IEEE International Conference on Computer Vision in 2019. The new version of the paper was published in the top journal in the field of artificial intelligence - IEEE TPAMI in 2020. The CCNet paper propose criss-cross attention (CCA) to effectively and adaptively model global context. CCA divides self-attention, which is the core of Transformer, into row-wise self-attention and column-wise self-attention. By applying this kind of sparse attention for two times, CCA has the ability of capturing full self-attention. It reduces the time/space complexity of self-attention / non-local network from O(N2) to O(N). In the scene segmentation problem in ADAS, CCNet is 6.5 times faster and reduces 91.4% memory cost with higher segmentation accuracy.
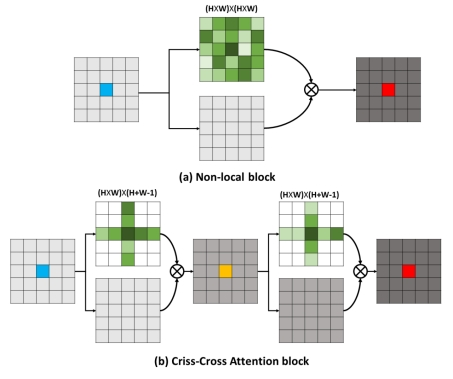
Figure 2: In CCNet recurrently performing two times criss-cross sparse self-attention (b) has the capacity of calculating one time dense self-attention (a).
The source code of CCNet has received over 1100 stars on Github and was incorporated into Axial Deeplab, the latest version of Deeoplab (the most advanced object segmentation framework launched by Google). It was also extended by the staff of UC Berkeley and Google Brain to Axial Transformer which deals with high-dimensional data, and was incorporated into MMSegmentation. CCNet has been published for more than one year, with over 600 Google citations and over 160 SCI citations for a single paper.